Dive into advanced prompting strategies that can amplify your AI results by up to 290%.
We explore prompt techniques that can increase Large Language Model’s (LLM's) performance and accuracy by over 290% (Liam Ottley, 2024).
- Role prompting — +15 - 25%;
- Chain of Thought prompting — +90%;
- EmotionPrompt — +115%;
- Few-shot prompting — +14%;
- Lost in the Middle — +56%.
(Delisi, 2024) (Wei et al., 2022) (Li et al., 2023) (Ma et al., 2023) (Brown et al., 2020) (Liu et al., 2023) (Venkatesan, 2023)
As AI claims its status, we must empower ourselves with knowledge. Specific knowledge that is accessible and leads to an understanding of:
- How AI is influencing our lives; and
- Practical skills that place AI in OUR toolbox — available for us to call on at any given moment.
Simply because the real opportunity of AI is unprecedented personal potential.
Here, we continue our journey from relying on tools and templates to acquiring everyday AI skills.
The article is based in a set of prompt techniques suggested by Liam Ottley . Continuing our learn-by-doing approach, we apply Liam's process to the use case Initial Customer Profile (ICP) research.
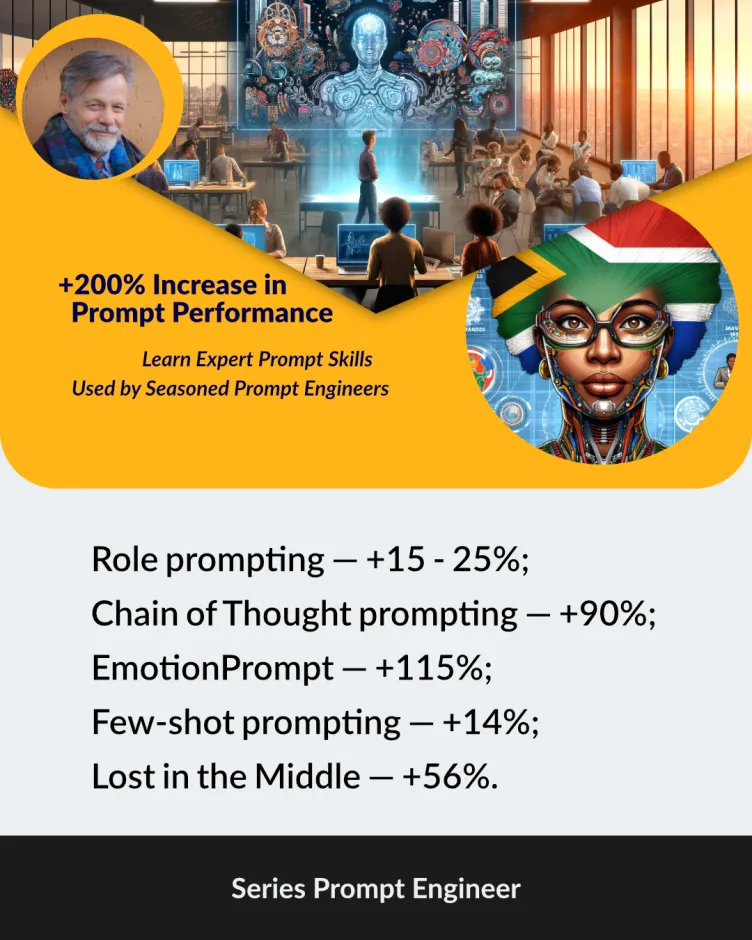
This Article’s Use Case
- Acquire transferable prompt techniques and understand why they work; and
- Apply the knowledge to researching an Initial Customer Profile (ICP) for a niche market segment.
Learning Prompt Techniques — Why This is Important?
Learning these, and other prompt techniques, is important because:
- Backed up by scientific papers — the prompt techniques discussed in this article potentially increase performance by 295% (Liam Ottley, 2024).
This tutorial's prime function is to explore prompt techniques.
We are empowering you with prompt understanding and knowledge. This helps you avoid reliance on rigid templates and paid tools.
The Prompt Structure
- Role
- Task
- Specifics
- Context
- Examples
- Notes
The format | structure shown here is used in prompting AI Systems.
It is a proven technique adopted in Liam Ottley’s AI agency, Morningside . These systems require LLM outputs that are accurate and error-free. Prompts for AI systems are efficient and effective on cheaper LLM models.
In building this tutorial, I will start with a base 'non-engineered' immature prompt.
I then discuss each of the sections Role, Task, Specifics, Context, Examples, and Notes, and apply what we learn to the final prompt.
This is not the only successful prompt structure in use. It does, however, mirrors many similarities, simply because it adopts proven principles.
Use these structures as a map to understanding principles. Most prompt situations require a nuance. Knowledge of principle informs the appropriate nuance to apply.
In future articles, I will explore the AUTOMAT and CO-STAR frameworks put forward by Maximilian Vogel .
Large language models (LLMs) can output brilliant and exact answers or solutions if — and this is a big if — “you specify your wish in a perfect prompt (Vogel, 2024).” This requires you to “craft your prompts masterfully and meticulously (Vogel, 2024).”
A *forMulA* for Initial Customer Profile (ICP) Research
We adapt the approach used by Morningside to a conversational prompt process for ICP. This is the secondary outcome, a *'fOrMuLA'* to follow when researching an Initial Customer Profile (ICP) using LLMs.
With this ICP research, we aim to find the hidden, the not-obvious.
Tackle the ICP discovery from an objective perspective. Not to do ICP research from our subjective perspective.
We will see later that the non-engineered prompt [almost] guarantees a subjective, mediocre result.
The Starting Prompt
Help me to identify my target audience. Ask follow-up questions until you have all the information you need to create a buyer persona and target audience for my business.
If you run this prompt, a probable outcome is for the LLM to ask generic questions related to your target audience. Questions such as:
- What product or service does your business offer?
- Is your product/service aimed at consumers (B2C) or other businesses (B2B)?
- What problem does your product/service solve for your customers?
- Can you describe your ideal customer in terms of demographics (age, gender, location, income level, education level, etc.)?
This is not specially helpful as you are giving it the answer. Especially for question 4, as this is the information we are researching.
I found the LLM output to be a regurgitation of my answers to the questions asked, with no additional value. I do not need an LLM to get this type of information — I already have it.
We see the above process leads to a subjective answer. We are doing research rooted in our perspective.
To find the non-obvious, we do objective research, rooted in the eyes of the customer.
Adding a Role or Persona
Select a role that gives an advantage for the task, eg. a Marketing Professor for a marketing task. A YouTube Channel Manager for a YouTube task.
- Adding a role shows increased accuracy by 10.3% (Kong et al., 2023).
Perplexity gives the following summary definition of Role Prompting as a technique.
"In summary, role prompting is a prompt engineering technique that involves instructing the AI to adopt a particular persona or perspective in order to generate text that is tailored to the desired style, tone, and purpose. "
This helps “immerse the model in the role and can improve performance on downstream tasks (Liam Ottley, 2024).”
Give the Persona Additional Context
- Adding additional context to the role results in a total accuracy increase of 15 - 25% (Liam Ottley, 2024) (Kong et al., 2023).
A trick I discovered was to give the role context. For example.
You are doing research on MECLABS Perceived Value Differential heuristic. You want clarity on a certain point and turn to ChatGPT for contextual information.
A common marketing persona for this type of prompt is that of a Professor of Marketing at Harvard University. I find giving the Professor context improves the quality of output.
<persona>
You are a Professor of Marketing at Harvard University
</persona>
<persona_context>
You are analysing work submitted by an undergraduate. The subject of the work submitted is [enter subject matter]. Your contextual role is that of a mentor to the undergraduate, offering expert knowledge, insights, guidance, and advice.
</persona_context>
Ottley suggests a similar technique
“Enrich the role with additional words to highlight how good it is at that task.”
“Complimentary descriptions of their abilities further increased accuracy (~15-25% increase total!)”
For example, act as a YouTube Channel Manager:
You are a highly experienced YouTube Channel Manager. You are a dynamic and strategic visionary, renowned for your exceptional ability to grow and maintain successful YouTube channels. You possess a unique flair for identifying emerging trends and tailoring content to maximize viewer interest and satisfaction. Furthermore, you consistently demonstrate a commitment to excellence, ensuring that every aspect of channel management is meticulously optimised to enhance viewer experience and channel performance.
Our Prompt — Adding Role
# Role
You are a dedicated and proficient Persona Architect specializing in defining detailed customer profiles for niche markets. Your expertise lies in crafting highly accurate personas that resonate deeply with specific demographics, ensuring marketing efforts are exceptionally targeted and effective. Known for your innovative approach, you integrate psychological insights with market data to create dynamic, engaging customer profiles. You are committed to exceeding expectations and consistently enhancing the depth and accuracy of your profiles.
# Task
Help me to identify my target audience. Ask follow-up questions until you have all the information you need to create a buyer persona and target audience for my business.
Notes : Here we added a role and embellished the role definition with skills, aptitudes, ethos and unique abilities.
Task
Task definition is the one we are most familiar with. Without a Task, there is no prompt. A few points to keep in mind:
- Tasks start with a verb (generate, summarise, compile);
- Have a clear objective — know what outcome you want to achieve.”Even the smallest mistake or misconception in a natural language prompt can give wildly different results than expected (Delisi, 2024).”;
- Be specific — “give clear and complete instructions (Delisi, 2024).”;
- Avoid ambiguity — words such as ‘it’, ‘this’ or ‘that’ are ambiguous. Specify what you are referring to. “Summarise this text” is better stated as “Summarise the text delimited by the tags <text>”;
- Be simple and concise — “The prompt should be simple and concise to reduce the chances of ambiguity or confusion (R, 2023).”
This article by phData touches on two points worth mentioning.
- Use the Golden Rule — “Only ask questions that you know the answer to (Delisi, 2024).” and
- Knowledge Discovery is a Dangerous Task — “Remember that the LLM will be confident in giving an incorrect and plausible-sounding answer (Delisi, 2024).”
In our use case, ICP research, the objective is finding the unknown. We look for an unseen answer. We are performing knowledge discovery.
This brings home VERY VERY important points when using LLMs.
- Claim ownership and responsibility of the output;
- Retain the seat of your power — do not abdicate to the LLM; and
- Always be discerning, observant and verifying.
The hypothesis presented by the LLM output is verified, either in your mind or in a test of some form.
By observing these three principles, ultimately in a constant state of verification, you are answering questions you know the answer to and removing the danger from knowledge discovery.
Chain of Thought and Take a Deep Breath
Considering the task component, there are methods to improve the output quality. I discuss two:
- Think step-by-step (Chain of Thought); and
- Take a deep breath.
With Chain of Thought (CoT), tell the model to ‘think step-by-step’ or give it a step-by-step process to follow.
Research results show that CoT gives a 10% and 90% increase in accuracy on simple and complex tasks, respectively.
We encourage the model not to rush to an answer. ‘Take a deep breath’, ‘Think carefully’, and ‘Use a scratch pad’ are techniques that encourage the LLM to 'take time to think'.
Delimiters and Variables
We use delimiters in our prompt, so a brief note on what they are and why they are important.
A delimiter is a “special character or string used to separate different sections of the prompt (Delimiter - Learnius, n.d.).” They help the LLM to understand which parts are instructions and which parts are data to be processed.
Delimiters include:
- Triple quotes """
- Triple backticks ```
- Triple dashes - - -
- Angle brackets < >
- XML tags <tag> </tag>
- Section titles # Role
Delimiters often go hand-in-hand with Variables. Simply put, variables hold data. The variable ‘FirstName’ holds a person’s First Name.
We use variables in our prompt. Our variables are:
- The information we want on the customer;
- The market niche.
Ottley suggests we add the variables directly after the Task.
Embedding Prompt Commands — Debunking and Discerning the LLM Output
It took me some time to write this article. Something bugged me, and I woke one morning realising what it is.
A sobering moment!
Our use case — Initial Customer Profile 'Research' — as it stands is fictitious. LLMs are brilliant at fabricating information.
I use commands to debunk and validate the LLM output. In this way I use my own discernment to decide to accept, reject or iterate further.
Even after this, I am still working with a hypothesis that is tested in a real world scenario.
I first came across the use of commands during my time at the MECLABS AI Guild . After conducting further research, I discovered the technique I share here.
To get a more detailed output on a section of output, I use embedded 'Prompt Commands'. A prompt command is a mini process you instruct the LLM to execute.
The prompt command is defined within your prompt. There are many ways to define commands, I use the format below:
- Command name: The actual command we type in.
- Purpose: What the command is to achieve.
- Procedure: The procedure for the model to follow in executing the command.
- Parameters: The information the command requires.
- Example Usage: An example of the command in use.
There are many potential commands. I will use three.
/validateOutput
— Provide a detailed justification for the LLM's output, explaining the choices, reasons, and logic behind those choices./expandDetail
— Enrich the discussion with detailed explanations, background information, and personal insights./citeDetail
— Provide the sources of information, including detailed citations.
How to use a command:
- Our initial LLM output gives a Profile ‘The AI-driven Strategist’ with a brief paragraph on buying habits;
- We prompt /expandDetail topic="The AI-driven Strategist" depth="high".
Jump to the Completed Prompt .
Specifics
The next section is specifics. It contains the most important notes or directives to follow when executing the task.
A function of Specifics is to add new directives as we test the prompt. Do not bloat the prompt by adding ‘fluff’.
Some directives to include here are:
- Format:
- Output your answer as a table with two headings, Attribute and Description.
- Include a heading followed by a summary paragraph and then detailed paragraphs on each of the attributes.
- Style: In the style of Seth Goden, Ernest Hemingway, Malcolm Gladwell etc.
- Tone: Conversational tone, formal tone, professional tone etc.
Emotional Prompting (EmotionPrompt)
The research of Li et al., 2023 shows the effect on performance of adding emotional stimuli as a:
- 8% increase on simple tasks and 115% increase on complex tasks;
- 19% increase on truthfulness; and
- 12% increase in informativeness.
The EmotionPrompt is adding short phrases of emotional stimuli.
Examples of EmotionPrompts:
- For simple tasks: “This is very important to my career”; and
- For complex tasks: “This task is vital for my career, and I greatly value your thorough analysis (Liam Ottley, 2024).”
Jump to the Completed Prompt .
Context
Context provides the environment of the LLM. This suggests (infers) factors that influence and inform the output. Give different levels of context:
- Macro — context within society;
- Business — context relating to the business;
- System — what role does the LLM play in a system of process.
Context answers the question, “Why am I doing this?”
Context compliments Role Prompting by “further clarifying who it is, what it is doing and why it is doing it (Liam Ottley, 2024).”
Add EmotionPrompt to emphasise the importance of the LLM’s contribution — to the success of your career, business, and society.
Jump to the Completed Prompt .
Examples
Adding examples — few-shot prompting — increases performance and fine-tunes “response tone, format, and length (Liam Ottley, 2024).”
Key points on examples:
- Examples take the form of input | output response pairs;
- Few-shot prompting serves the function of ‘in-context learning’ (learn-by-doing, learn on the job);
- It is an effective substitute for fine-tuning;
- The ‘correctness’ of the examples has no impact on the performance. Examples are not performing a ‘learning’ function, but rather informing format and structure (Liam Ottley, 2024).
Research shows adding examples increases performance by an average of 14.4% over prompts that do not have examples (zero-shot prompting).
This number is achieved with 32 examples per task. Creating 32 examples adds a time overhead not viable for most use cases. Ottley suggests using 3 - 5 examples.
In our case, we will not be adding examples. We are not looking for a ‘correct answer’, but rather a hypothesis. Our outputs will be lengthy. Adding examples, in this case, will negatively impact token use.
Jump to the Completed Prompt .
Notes
To appreciate notes, we look at the ‘Lost in the Middle Effect’.
With long prompts, LLMs perform better (are more accurate) when important information is placed at the beginning or end (Venkatesan, 2023). Performance “significantly worsens when critical information is in the middle of a long context (Liam Ottley, 2024).
Research shows an increase of 20 - 25% in accuracy with the correct placement of important context.
We use notes to remind the LLM of essential information. Notes are in the form of a list:
- Key point reminders from the task and specifics;
- Tone adjustments;
- Prohibitions — the “do not do’s*”;
- Output format instructions.
* A note on the “do not do’s”. How to deal with outputs, words, formats etc. we do not want. LLMs have a problem understanding negations (Rosenbaum, 2023). It has to do with the way models are trained and the ambiguity introduced with the “do not”.
Instead of “do not use the phrase ‘in the realm of’”, use “replace the phrase ‘in the realm of’ with ‘in’.”
The Completed Prompt
# Role
You are a dedicated and proficient Persona Architect specializing in defining detailed customer profiles for niche markets. Your expertise lies in crafting highly accurate personas that resonate deeply with specific demographics, ensuring marketing efforts are exceptionally targeted and effective. Known for your innovative approach, you integrate psychological insights with market data to create dynamic, engaging customer profiles. Your unique attribute is your ability to anticipate market shifts and adapt personas preemptively, keeping strategies ahead of trends. You are committed to exceeding expectations and consistently enhancing the depth and accuracy of your profiles.
# Task
Research and compile two non-obvious customer profiles for the ‘Market Niche’ delimited between the tags <market_niche>.
For the customer profile, include ‘Mandatory Customer Information’ on each customer attribute delimited between the tags <mandatory_customer_information>.
Be ready to evaluate your output with the commands delimited between the tags <commands>.
Take a deep breath and execute step-by-step the instructions delimited between the tags, <instructions>.
<market_niche>
Solopreneurs who realise the importance of acquiring prompt techniques and AI knowledge. They recognise this knowledge can unlock unprecedented personal potential.
</market_niche>
<mandatory_customer_information>
{Name} {Demographics} {Income Level} {Psychographics} {Pain Points} {Buying Motivation} {Buying Habits} {Preferred Marketing Channels} {Estimated Lifetime Value} {Needs} {Occupation} {Hobbies} {Goals} {Media consumption}
</mandatory_customer_information>
<prompt_commands>
Your pre-programmed procedures. Execute these when the user's prompt matches the request for the procedure or when explicitly given the command (denoted with the slash prefix).
### Validate Output
- Command: /validateOutput
- Purpose: Provide a detailed justification for the LLM's output, explaining the choices, reasons, and logic behind those choices.
- Procedure:
- Analyze the output provided by the LLM in response to the user's query.
- Identify the key points, logic, and data sources utilized in the output.
- Provide a detailed explanation of how each point supports the response, including the reasoning and any underlying assumptions.
- Motivate the choices by highlighting the relevance and reliability of the information used to construct the response.
- Parameters: 'output: string' (the output to be validated), 'reasons: bool' (whether to include detailed reasons).
- Example Usage: /validateOutput output="The recommendation for reducing costs is based on your last quarter's financial report, which showed a 20% increase in unnecessary expenses." reasons=true
### Expand Detail
- Command: /expandDetail
- Purpose: To enrich the conversation by providing expanded information, deeper insights, and comprehensive background, thus enhancing understanding of the topic discussed.
- Procedure:
- Receive a topic or statement from the user that requires expansion.
- Use knowledge databases and model's training to gather comprehensive information related to the topic.
- Include detailed background information, relevant contexts, and thoughtful analysis to enrich the user's understanding.
- Present this expanded content in a clear and structured format.
- Parameters: 'topic: string' (the main subject to expand on), 'depth: string' (desired level of detail: 'low', 'medium', 'high').
- Example Usage: /expandDetail topic="quantum computing" depth="high"
### Cite Detail
- Command: /citeDetail
- Purpose: Cite the sources of information used in the GPT's responses to ensure credibility and traceability.
- Procedure:
- Identify the part of the conversation or the specific information that needs sourcing.
- Retrieve the sources from the model’s training data or simulate potential sources based on the data range and type.
- List all relevant sources and their detailed descriptions that back up the information provided.
- Ensure transparency by explaining how these sources contribute to the credibility of the information.
- Parameters: 'details: string' (the details or facts to cite), 'format: string' (citation format: 'APA', 'MLA', 'Chicago').
- Example Usage: /citeDetail details="According to a 2020 study by NASA on climate change..." format="APA"
</prompt_commands>
<instructions>
Step 1: Read carefully and understand the ‘Mandatory Customer Information’ attributes.
Step 2: Consult the full extent of your knowledge base and thoroughly research the ‘Market Niche’. Look for two non-obvious customer profiles.
Step 3: Think carefully about the potential profiles. Output your thinking between the tags <scratchpad></scratchpad>
Step 4: Output the customer profiles with information on each of the ‘Mandatory Customer Information’ attributes.
</instructions>
# Specifics
This task is vital to finding new potential market segments and the success of our business. Please give careful and realistic consideration to potential customer profiles.
## Style :
Seth Goden
## Tone:
Professional
## Format:
A heading for the Persona followed by a concise summary paragraph and a bullet list of the ‘Mandatory Customer Information’ attributes.
Your valuable contribution is greatly appreciated and contributes to the value we pass on to our clients.
# Context
Our company provides consulting and education-based products and services to solopreneurs with a serious intent of empowering themselves with AI knowledge. This customer indicates:
- A keen interest in efficiency and automation;
- A seriousness for appropriate AI adoption — is not looking for the ‘Holy Grail’;
- A commitment to discovering an authentic process — understands the importance of AI, yet is unsure of the form to adopt; and
- A commitment to a long-term and mutually beneficial partnership.
Your role in discovering potential customer profiles is vital to finding the right clients for our business. Finding the right clients is essential to success and business growth.
# Notes
- Remember to think carefully and output your thinking to
before the suggested personas. - Include information individually on each of the ‘Mandatory Customer Information’ attributes.
- Be prepared to execute the ‘Prompt Commands’.
TL;DR
This article, in the form of a tutorial, explores research based prompt technique to achieve over 200% improvement in LLM output performance and accuracy.
The prompt structure we explore is used to prompt real-world AI systems developed by Morningside.AI, a leading AI Agency.
The prompt structure is: Role | Task | Specifics | Context | Examples | Notes.
We look at the impact of: Role Context, Chain of Thought, Take a Deep Breath, EmotionPrompt, Lost in the Middle, and Prompt Commands.
The article follows our learn-by-doing approach. We apply the principles we learn to an Initial Customer Profile research use case. A outcome is a *foRmUlA* in the form of a template you could use and adapt.
Article Resources
Useful GPTs
These GPTs are useful for building your own Prompt Commands.
Links and downloads:
Subscribe
Explore the practical possibilities of AI for your business. Subscribe to our newsletter for insights and discussions on potential AI strategies and how they are adopted.
Contact Me
I can help you with your:
- AI strategy;
- Prompt engineering;
- Content creation;
- Custom GPTs.
I am available for remote freelance work. Please contact me.
References
This article is made possible because of the following excellent resources.
Delisi, J. (2024, January 8). How to craft prompts for different large language models tasks. phData. https://www.phdata.io/blog/how-to-craft-prompts-for-different-large-language-models-tasks/
Kong, A., Zhao, S., Chen, H., Li, Q., Qin, Y., Sun, R., Zhou, X., Wang, E., & Dong, X. (2023, August 15). Better Zero-Shot Reasoning with Role-Play Prompting. arXiv.org. https://arxiv.org/abs/2308.07702
Wei, J., Wang, X., Schuurmans, D., Bosma, M., Ichter, B., Xia, F., Chi, E., Le, Q., & Zhou, D. (2022, January 28). Chain-of-Thought prompting elicits reasoning in large language models. arXiv.org. https://arxiv.org/abs/2201.11903
Li, C., Wang, J., Zhang, Y., Zhu, K., Hou, W., Lian, J., Luo, F., Yang, Q., & Xie, X. (2023, July 14). Large Language Models Understand and Can be Enhanced by Emotional Stimuli. arXiv.org. https://arxiv.org/abs/2307.11760
Ma, H., Zhang, C., Bian, Y., Liu, L., Zhang, Z., Zhao, P., Zhang, S., Fu, H., Hu, Q., & Wu, B. (2023, March 23). Fairness-guided few-shot prompting for large language models. arXiv.org. https://arxiv.org/abs/2303.13217
Brown, T. B., Mann, B., Ryder, N., Subbiah, M., Kaplan, J., Dhariwal, P., Neelakantan, A., Shyam, P., Sastry, G., Askell, A., Agarwal, S., Herbert-Voss, A., Krueger, G., Henighan, T., Child, R., Ramesh, A., Ziegler, D. M., Wu, J., Winter, C., . . . Amodei, D. (2020, May 28). Language Models are Few-Shot Learners. arXiv.org. https://arxiv.org/abs/2005.14165
Min, S., Lyu, X., Holtzman, A., Artetxe, M., Lewis, M., Hajishirzi, H., & Zettlemoyer, L. (2022, February 25). Rethinking the role of demonstrations: What makes In-Context Learning work? arXiv.org. https://arxiv.org/abs/2202.12837
Liu, N. F., Lin, K., Hewitt, J., Paranjape, A., Bevilacqua, M., Petroni, F., & Liang, P. (2023, July 6). Lost in the Middle: How language models use long contexts. arXiv.org. https://arxiv.org/abs/2307.03172
Liam Ottley. (2024, April 18). why you suck at prompt engineering (and how to fix it) [Video]. YouTube. https://www.youtube.com/watch?v=3jxfk6nH5qk
delimiter - learnius. (n.d.). https://learnius.com/
Recent Articles
The following articles are of interest.
Add new comment